Intelligence change vs. climate change
Differences in long-term vision, time horizon & speed of change
“AI is no different from climate. You can’t get safety by just having one country or a set of countries working on it. You need a global framework. (…) There is concern that we could bifurcate here but I think it's important not to do so. I'm optimistic because just like in climate I think there's more alignment. We have things like the Paris agreement. The world comes together because everyone shares the climate of the Earth. I think that's true for AI. So, down the line I think that we there will be a common gravitational pull, regardless of who you are, to try and converge.” – Sundar Pichai, 2020
“We need the AI researchers to reach a consensus, in much the same way as climate scientists have reached a consensus on climate change, because politicians and other decision makers are gonna be looking for technical opinions from the AI researchers but if the AI researchers have all sorts of different opinions, then they’re gonna be able to pick and choose whatever suits them.” – Geoffrey Hinton, 2023
“I mean if you told me we had 20 years to get it right, you know, 30 years, 50 years… I mean climate change, heck, we're eventually going to get there, we'll get to net zero, we'll have the new technologies. You know, at the cost of a lot of species and a lot of human beings, but we will eventually get there. We don't have climate change time on AI. We can't get it wrong for that long. We can't ignore it for that long. We can't let vested interests control the outcomes for that long and that means that we need hybrid state and private sector governance on this yesterday.” – Ian Bremmer, 2023
1. Introduction
This text explores the analogy between the rise of AI and climate change.
Section 1 highlights how the analogy is used and how the domains of AI and climate change interact with each other.
Section 2 analyzes five key structural commonalities: 1) Complexity, 2) trend and hazards, 3) global public goods, 4) powerful private actors, and 5) concerns about existential risk.
Section 3 highlights five key structural differences: 1) scientific consensus, 2) system-orientation, 3) “wizard” vs. “prophet” vision, 4) time horizon, and 5) speed of change.
1.1 How the analogy is used
Sundar Pichai: The CEO of Google has repeatedly referred to climate change and the Paris Agreement as a model for a global governance response to AI, highlighting that it is a global challenge that will need an international agreement across the geopolitical divide (2018, 2018, 2020, 2021, 2023, 2023).
IPCC for AI: Various researchers and policymakers have called for an equivalent of the Intergovernmental Panel on Climate Change (IPCC) for AI. In other words, an international panel that summarizes the state of science and help policymakers understand what the scientific consensus on the trajectory of “intelligence change” is. There are three main intergovernmental efforts along this line, which re discussed in more depth in the article “IPCC for AI”:
In 2019, France and Canada have launched an IPCC-inspired Global Partnership on AI and subsequent observatory efforts at the OECD
In 2023, through the Bletchley track of AI safety summits initiated by the UK about 30 countries have agreed to the development of an International Scientific Report on the Safety of Advanced AI
In 2024, the United Nations plan to have an International Scientific Panel on AI and Emerging Technologies to conduct scientific risk and opportunity assessments in the draft of the Global Digital Compact.
1.2 Literal overlaps
AI for climate change solutions: AI can help to fight climate change with applications in area such as climate modeling and energy efficiency. Rolnick et al. (2019) have assembled a long list of areas where AI might have some positive potential.
The hope that AI can help to tackle climate change is often repeated by tech leaders and included in governmental AI strategies (e.g., EU Coordinated Plan, UK AI Strategy). Probably the most widely cited real-world example of a positive environmental impact of AI has been Google DeepMind using AI to optimize the cooling of a data center. The company trained an AI system on thousands of sensors in its data center in Singapore to predict its short-term cooling needs and managed to reduce the energy required for cooling by 40%. Cooling represents about 30-40% of an average datacenter’s overall energy consumption. The idea is to move towards autonomous data center cooling and industrial control.
AI as a contributor to greenhouse gas emissions: Some argue that big tech offering its AI services to help fossil fuel industries find new oil fields and to automate oil drilling may prolong the energy transition.
More importantly, AI’s hunger for electricity is growing exponentially. Despite nice successes such as DeepMind’s efficient cooling, the energy consumption of AI datacenters is not decreasing, nor is it stable, instead, it is increasing exponentially. GPUs already consume more energy than most countries, and their energy consumption is set to double by 2026.
Net zero goals as a potential constraint on datacenter growth: Most large economies plan to achieve net zero emissions to address climate change. At the same time, the war in Ukraine and the phase-out of internal combustion engine cars already put stress on the electric grid in Europe. European countries have run extensive campaigns to ask their citizens to reduce their energy consumption and heating. Hence, not everybody is happy to build power plants that could power entire cities of humans to build new datacenters. The Netherlands, where environmentalists have repeatedly clashed with big tech over datacenter expansion plans, may to some degree be a microcosm of the shape of things to come.
On the other end of the spectrum, Leopold Aschenbrenner argues that the US should abandon its climate commitments if they slow down the buildup of more AI datacenters: “The barriers to even trillions of dollars of datacenter buildout in the US are entirely self-made. Well-intentioned but rigid climate commitments (not just by the government, but green datacenter commitments by Microsoft, Google, Amazon, and so on) stand in the way of the obvious, fast solution. (…) I’d prefer clean energy too—but this is simply too important for US national security.”
2. Key Commonalities
2.1 Complexity
Both climate change and the long-term rise and impact of AI are characterized by high complexity and significant uncertainty. The basic reason for this is that both depend on global anthropogenic activity, meaning if we would want to predict the exact amount of greenhouse gas emissions or the exact amount of AI compute in two decades, we would strictly have to model the entire world economy as an open, complex system. On top of that, both the global climate and the rise of AI contain feedback loops, non-linearities, and threshold effects, which means the system may go through critical transitions that cause abrupt shifts.
Some have argued that both climate change and AI qualify as “wicked problems”, a class of issues, which are ill-defined, lack a clear stopping rule and have no simple yes or no answers. Similarly, some have called them “super wicked problems”, adding that there is a pressing deadline to finding a solution, no central authority dedicated to finding a solution, that those seeking to solve the problem are also causing it and that certain policies would irrationally impede future progress.
2.2 Trend and hazards
Climate: Climate change is a global long-term trend. It is not a hazard in the sense that it is not a discrete event with a specific amount of costs and deaths in a specific area. Insurances don’t offer coverage against climate change, they offer to cover the risks from a list of specific hazards, such as storms, floods, heatwaves, or wildfires. However, climate change as a long-term trend changes the frequency, distribution, and intensity of weather-related local hazards.
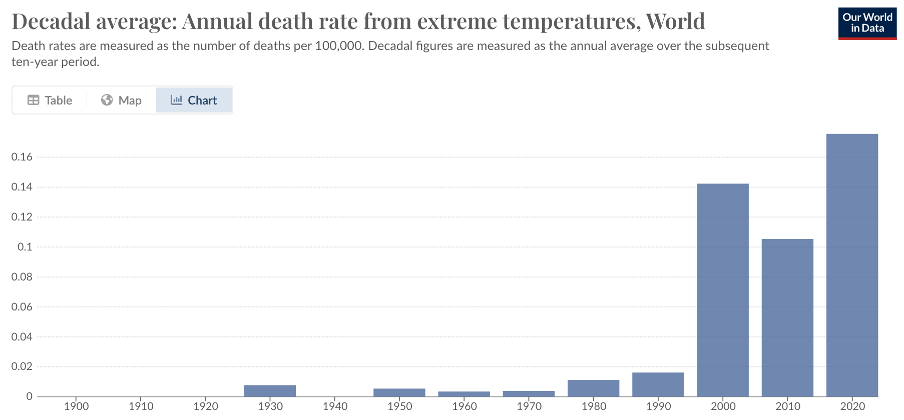
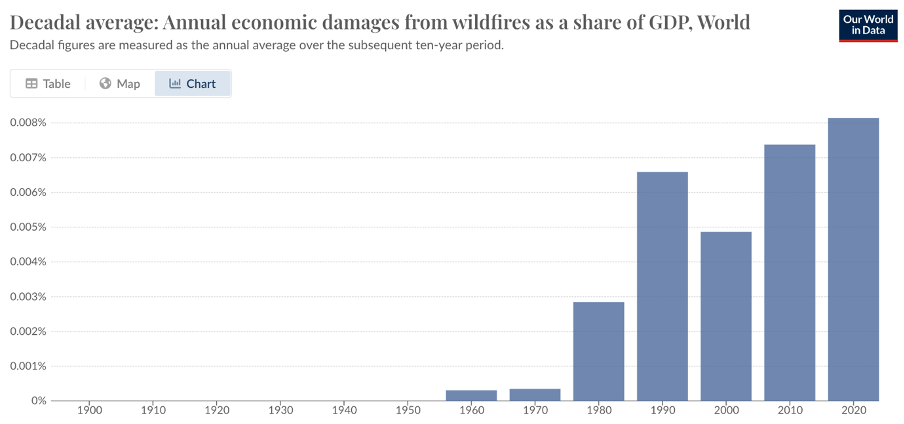
AI: It can make sense to think about some AI risks the same way. There is a longterm trend of the rise of AI. This is a trend and not a hazard. However, having ever more powerful AI ever more deeply embedded into every aspect of our economy and our lives means that the dependencies on and the risk surface of AI systems grow over time.1
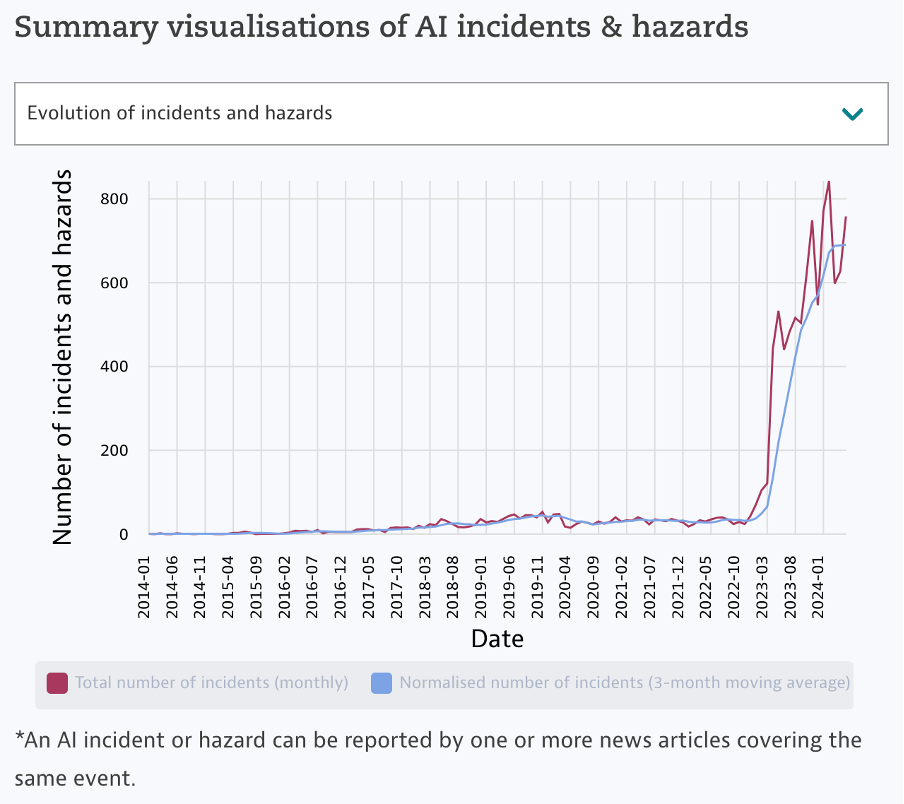
This framing is not adequate for all aspects of AI governance, but it can still be useful. In climate change, there is no division between a “short-term weather risk” community and “long-term weather risk” community because the issue is framed centered on the long-term trend, which creates short-term and long-term risks. Hence, people worried about hurricanes or wildfires and those worried about runaway climate change still see each other as allies in arguing for more climate change mitigation and adaptation rather than as competitors for attention.
In contrast in AI policy there is sometimes the tendency to divide the 15% that don't lobby for big tech into camps. The “short-term camp” thinks that speculative future harms should not distract from already occurring harms. The “long-term camp” thinks that almost all current harms are a distraction and that the only thing that matters for the future of civilization are the risks from superintelligence. A framing that focuses more on the underlying long-term trend rather than current or future events, and highlights that some of the problems that we face today are in some ways miniature challenges of future AI challenges might offer more common ground.
2.3 Global public goods
“You just can't solve climate change or regulate AI on the level of a single nation. So, the only solution to these global problems, is greater global cooperation.” – Yuval Noah Harari, 2018
Climate: When a country conducts economic activity that emits greenhouse gases, the benefits of that economic activity accrue locally, whereas the negative effects of the global warming caused by greenhouse gases—such as more wildfires or heatwaves—are distributed globally. Countries can internalize the externalities associated with carbon emissions by assigning a cost to emitting carbon dioxide.
Reducing greenhouse gas emissions is an aggregate effort global public good. Without global coordination, individual countries might have little incentive to reduce emissions, as the benefits of their actions (reduced global warming) are shared globally, while the costs (economic and social adjustments) are borne locally. Meaning countries may be incentivized to attempt to free ride on the efforts of others.
AI: Not everything in AI is a global challenge. Countries have their own regulations for Internet content, such as hate speech, bias, or adult material. Hence, some level of shallow Internet fragmentation along political borders was arguably inevitable2 and it seems highly likely that countries will also want to have their own rules with regards to appropriate AI content. For example, the US discourse around algorithmic bias is captured by the idea that minorities are underrepresented in datasets and that this lower legibility puts them at a disadvantage in the provision of public services. However, globally, many minorities face repression from their governments, and increasing the legibility of minorities to the state is not in their interest. For example, the Uighurs are not underrepresented in Chinese facial recognition datasets, they are massively overrepresented. Hence, a global agreement on algorithmic bias just doesn’t make sense.
In contrast to climate change, there is also no consensus that it would be desirable to limit intelligence change to a specific amount of overall computing power, and hence there is also no aggregate effort global public good in limiting or reducing it. However, there are aspects of the AI challenge that are indeed global. First, there is a mutual restraint global public good between frontier AI companies and great powers to avoid an arms race, to not hand over crucial military decisions over to AI (e.g., nuclear weapons), and to not develop and release an uncontrollable, unaligned superintelligence. Second, there is a weakest link global public good to ensure that criminals and terrorists are denied access to advanced AI that could be used to create serious harms (e.g. bioweapons).
2.4 Powerful private sector
Climate: The companies involved in extracting, refining and selling fossil fuels are amongst the largest and most powerful companies of the world. Shell, PetroChina, Chevron, Exxon Mobil, and Saudi Aramco all have more than 200 billion USD in market cap.
AI: As of writing this, 7 out of the top 10 most valuable companies in the world by market capitalization were tech, including the largest AI chip designer (NVIDIA), the largest AI chip manufacturer (TSMC) and the largest operators of AI datacenters (Microsoft, Alphabet, Amazon, Meta).
2.5 Concerns about existential risk
Climate: We are still far away from a runaway reaction that would turn Earth into Venus (for that you need to boil away the oceans, right now we are still expanding them by melting ice). However, there are much earlier tipping points for agriculture, and socioeconomic stability. There is no scientific consensus on how many degrees of global warming would constitute an existential threat to humanity. Existential concerns are part of the public discussion around climate change, as evidenced by movements such as the “extinction rebellion” whose declared aim it is to prevent the extinction of humans and all other species due to climate change.
AI: Based on a large sample of surveyed AI scientists, the mean estimated likelihood of AI creating an extremely bad future on par with human extinction is about 9% and the median estimate about 5%. In May 2023, more than a hundred leading Western and Chinese AI scientists, and the most important tech CEOs signed a joint statement on AI risk that states that mitigating the risk of extinction from AI should be a global priority.
3. Key Differences
3.1 Scientific consensus
Climate: The climate movement is smart to always emphasize the overwhelming scientific consensus that climate change is real and has been caused by humans.
AI: While there has been some progress in AI researchers making joint statements and some work towards an international scientific panel, there are still significant disagreements between leading AI researchers (e.g., there is a very wide range of “p(doom)” estimates).
Having said that, a part of the perceived difference is due to framing. Yes, climate science is grounded in much more mature modeling and the IPCC scenarios reflect a broad consensus. Still, the well-known agreement that 97% of scientists think that climate change is primarily caused by humans is not that meaningful. There is less scientific agreement, when it comes to the severity of climate change over different time horizons and what we should do about it.
Asked differently, is there a single AI scientist that denies that there is a manmade change in the composition of intelligence on Earth? If we take the popular sport of predicting a specific date for “AGI”, the amount of divergence depends a lot on framing. 90% of surveyed AI experts expected that unaided machines can accomplish every task better and more cheaply than human workers within the next 100 years. That alone would seem like a sufficient justification to think, long and hard about the transition from a human-controlled future to an AI-controlled future.
3.2 System-orientation
Climate: As reflected in the naming of the field as climate science, rather than say “artificial energy” or “machine burning”, the focus is on the planetary-scale system. Climate science is a field of study that is led by independent academics and supported by global, largely public networks of sensors.
AI: As reflected in the naming of the field, the focus is on the level of individual technological artifacts. It is “artificial intelligence” and not “intelligence change” or “cybernetics”. Meaning the focus is on the (private sector) experts that build these individual artifacts. There are comparatively few individuals that monitor, measure, and project system-wide AI capabilities.
3.3 Wizard vs. prophet vision
The Wizard and the Prophet is a great book by Charles Mann that defines two archetypes for thinking about the future:
Wizard: Strong belief in science and technology to expand our boundaries and deliver abundance. We are not in a sustainable equilibrium, but we don’t need to be, as long as our technological capacity to produce and adapt grows fast enough.
Prophet: Strong belief that we need to respect nature and learn to live sustainably within planetary limits. Humans are burning through scarce natural resources. The only way forward is reducing our consumption of energy and materials.
This distinction can be a useful framework to think about problem constitution and risk perception in international affairs. Overall, prophets might be more concerned about most natural risks and risks related to sustainability than wizards because they mainly project consumption pattern forwards. In contrast, wizards might be more concerned about adversarial threats because the projected technological capacities make misuse and malicious use worse.3
Climate: The framing of energy is dominated by the prophet vision. Specifically, there is a broad consensus that we should reduce our carbon footprint and move towards net zero. Most large countries and large companies have bought into this vision. When thinking about the concrete means and solutions to achieve this overarching vision some prefer investments in science and technology, where others prefer a reduction of consumption. However, both groups concur on the overarching vision of reducing emissions. Only a small minority outside of the mainstream argues that humanity’s ability to adapt will continue to outpace climate change for the foreseeable future and that large-scale geoengineering should be the default plan rather than an emergency option.
The following is a visualization of archetypal positions (Epstein4, Gates5, Sandberg6, Thunberg7) in the climate debate in a wizard vs. prophet matrix.
Prophets did not always dominate energy policy. This is a shift that happened around 1970. Before that, it was mainstream science8 and mainstream science-fiction to presume that humans will gain rather than lose control over the Earth’s climate.
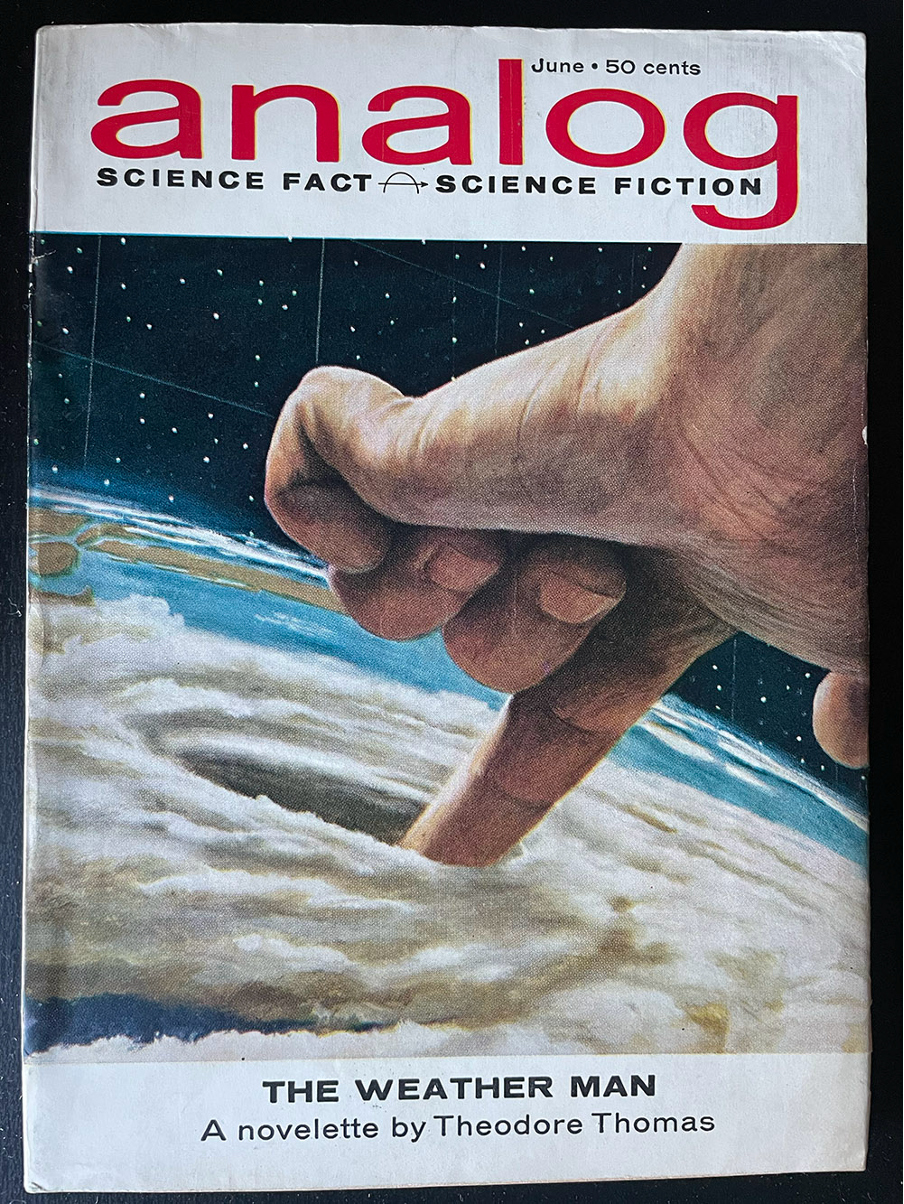
AI: In AI, the framing of intelligence is dominated by the wizard vision. This vision is not explicitly mentioned in strategies of countries and companies. Most actors do simply not have a long-term vision on AI. However, it is the logical trajectory in the absence of coordination, and it is how the AI debate is framed by those who are talking about the long-term future of AI. Most leading voices in potentially slowing down AI believe in a temporary prophet strategy, not a prophet vision. For example, a transhumanist Oxford prof with a cryonics contract is not exactly a “luddite”.
The following is a visualization of archetypal positions (Sutskever9, Huxley10, Ord11, Butler12) in the AI debate in a wizard vs. prophet matrix.
Either way, intelligence and energy are set for a clash. This doesn’t necessarily mean that the wizard vision or the prophet vision must completely break the other across both energy and intelligence, but it does create an interesting tension.
3.4 Time horizon
Climate: The Intergovernmental Panel on Climate Change makes regular in-depth assessments of climate scenarios until 2100 with some subchapters going as far as year 2300 and even year 3000.
This long-term thinking has also translated into concrete long-term goals and actions. There is an international agreement on a common long-term goal for climate change. Most major economies have adopted carbon neutrality targets (e.g., Germany by 2045, the EU, Japan, and UK by 2050, China by 2060, India by 2070) and this is not just talk. Hundreds of billions are spent every year in pursuit of strategies to achieve these goals (e.g., European Green Deal).
AI: The time horizon considered for the projection of intelligence change and AI policies is much shorter than those for climate change. This incoherent time horizon across issue areas allows policymakers to more or less sidestep the inconvenient truth that AI is set to dominate Earth civilization long before 2100.
There is no international scientific body that makes long-term projections about AI. For example the Interim Report from the International Scientific Report on the Safety of Advanced AI is primarily discussing past data points rather than forward projections of them.13 On the level of national AI strategies many do not have a clear time horizon. The furthest time horizons seem to be goals set about 10 years into the future (e.g. UK, China).
3.5 Speed of change
“Climate change has taken decades to be acknowledged and confronted; for AI, decades could be too long.” – Yoshua Bengio et al., 2024
Climate: Climate change typically operates at slow speed. Significant changes in global temperatures, sea levels, and atmospheric CO2 concentrations take decades to centuries to manifest.
Doubling periods
annual global greenhouse gas emissions: ca. 50 years
cumulative global greenhouse gas emissions: ca. 30 years
global average concentration of carbon dioxide (CO2) in the atmosphere: N/A - the global CO2 level has “only” increased by about 50% since the reference period (1850-1900, 280 ppm) so far. So, 150 years+
global average temperature: N/A, increase from 13.7°C (pre-industrial) to 14.8°C (today) to 16.4°C (projected based on current actions, 2100)).
Reporting periods
An updated synthesis report from the Intergovernmental Panel on Climate Change is published about every 5 years.
AI: AI development is characterized by exponential growth in capabilities and applications. The rapid pace of improvement means that AI technologies and their impacts can change dramatically in just a few years.
Doubling periods:
annual global production of AI hardware: less than 1 year
cumulative global production of AI hardware: less than 1 year.
global cumulative natural and AI computing power in the economy: ca. 50 years. The current doubling time is based on the doubling time of human population. Once AI hardware becomes dominant this accelerates to roughly match the cumulative global production of AI hardware.
Reporting periods
The zero draft of the Global Digital Compact foresaw a reporting period of the International Scientific Panel on AI of every 6 months.
I am not familiar with a disaster loss database for AI and maybe it’s still a bit too early for this. The OECD monitor seems largely automated based on news articles with a substantial rate of false positives. Still, I would read it as an imperfect indicator of the unfolding social ripple effects of generative AI.
Jack Goldsmith & Tim Wu. (2008). Who Controls the Internet?: Illusions of a Borderless World. Oxford University Press.
For example, Herman Kahn was the archetypal wizard of the nuclear age. He was very worried about the existential risk from nuclear war. However, any nuclear lobbyist that might have decried Kahn as a “luddite”, a “techno-pessimist”, or a “decel” would be confused. In fact, Herman Kahn is the author of “The Next 200 Years”, maybe the most significant techno-optimist response to the prophet-bestseller “Limits to Growth” in the 1970s.
“One of the key benefits of more fossil fuel use, I will argue, will be powering our enormous and growing ability to master climate danger, whether natural or man-made—an ability that has made the average person on Earth 50 times less likely to die from a climate-related disaster than they were in the 1°C colder world of one hundred years ago.” – Alex Epstein. (2022). Fossil Future. Penguin Books. p. 4
“1. To avoid a climate disaster, we have to get to zero. 2. We need to deploy the tools we already have, like solar and wind, faster and smarter. 3. And we need to create and roll out breakthrough technologies that can take us the rest of the way.” – Bill Gates. (2021). How to Avoid a Climate Disaster. Penguin Books. p. 8
Anders Sandberg et al. (2017). That is not dead which can eternal lie: the aestivation hypothesis for resolving Fermi's paradox. arxiv.org
“When it comes to the climate and ecological crisis, we have solid unequivocal scientific evidence of the need for change. The problem is, all that evidence puts the current best available science on a collision course with our current economic system and with the way of life many people in the Global North now consider their right. Limitations and restrictions are not exactly synonymous with neoliberalism or modern western culture.” – Greta Thunberg. (2022). The Science Is As Solid As It Gets. In: G. Thunberg (Ed.). The Climate Book. Penguin Books. p. 21
Here is Thomas Malone, the Chairman of the Committee on Atmospheric Sciences of the National Academy of Sciences (top advisory body to the US government on climate science at the time), in 1968: “Weather modification has reached a take-off point from which further progress will take place at an accelerating rate.“ Malone was also aware of greenhouse gas induced global warming, but he didn’t think that it would be likely that this would grow faster than our ability to control the climate: “A distinct probability should be recognized that large-scale climate modification will be affected inadvertently before the power of conscious modification is achieved. (…) There is a small probability that these efforts will not be tolerable.” Thomas F. Malone. (1968). Weather: Man Will Control Rain, Fog, Storms and Even Possibly the Climate. In: Toward the Year 2018. Foreign Policy Association. pp. 61-74.
“How do we ensure AI systems much smarter than humans follow human intent? Currently, we don't have a solution for steering or controlling a potentially superintelligent AI, and preventing it from going rogue. Our current techniques for aligning AI, such as reinforcement learning from human feedback, rely on humans’ ability to supervise AI. But humans won’t be able to reliably supervise AI systems much smarter than us, and so our current alignment techniques will not scale to superintelligence. (…) Our goal is to build a roughly human-level automated alignment researcher.” - Jan Leike & Ilya Sutskever. (2023). Introducing Superalignment. openai.com
The description of Brave New World as statist may seem confusing because the book contains future technology, a contrast with the “Savage Reservation” and pro-progress propaganda of the world state. However, objectively Brave New World is a statist society with an absence of robots and AI, a technologically frozen societal pyramid, tightly guarded forbidden knowledge, and a direction of all human energy towards hedonism and pseudo-innovation (“obstacle golf”). Aldous Huxley. (1932). Brave New World. Chatto & Windus.
There is a fairly broad consensus that it would be desirable to have time for AI interpretability, safety, and alignment to catch up with AI capabilities before irreversibly handing control over to AI systems. “The Long Reflection” is the extremized archetype of that, which argues that we should perhaps have a pause for “centuries” to reflect on the best way forward.
Samuel Butler. (1872). Erewhon: or, Over the Range.
The only exceptions: Compute trends are extrapolated 2 years to 2026, and there is a note that there might be a shortage of training data by 2030.